Understanding the Importance of Labeling Tool Machine Learning
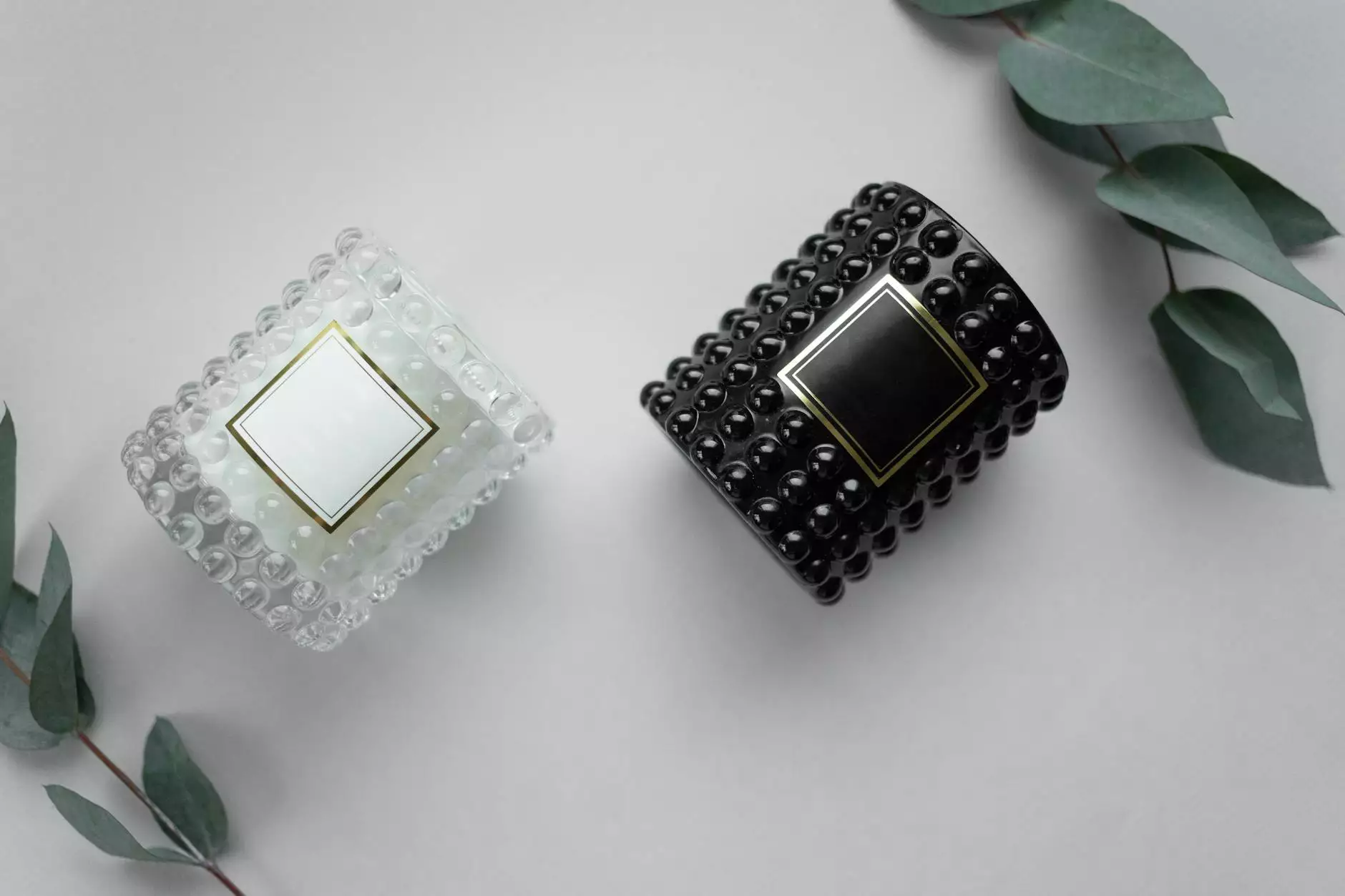
In the rapidly evolving landscape of data science and artificial intelligence, the need for efficient and precise data annotation cannot be overstated. As organizations increasingly rely on machine learning models to derive insights from vast datasets, the role of labeling tools becomes crucial. This article explores the integration of labeling tool machine learning within data annotation, shedding light on its benefits, applications, and future prospects.
The Fundamentals of Data Annotation
Data annotation is the process of marking up data to make it suitable for training machine learning algorithms. This involves identifying and labeling various data elements, such as images, videos, and texts, enabling algorithms to recognize and learn patterns effectively. Here are some reasons why data annotation is pivotal:
- Contextual Understanding: Properly labeled data helps algorithms understand context and make informed predictions.
- Improving Model Accuracy: Accurate annotations lead to more robust models, reducing the likelihood of errors in predictions.
- Variety of Applications: Annotated data is essential in various sectors, including healthcare, finance, and autonomous driving.
The Evolution of Labeling Tools
Traditionally, data annotation was a manual process, labor-intensive and time-consuming. However, the advent of machine learning technologies has revolutionized this domain. Here's a brief overview of how labeling tools have evolved:
- Manual Annotation: Human annotators read and label data, prone to inconsistencies.
- Automated Annotation: Initial attempts to automate the process using simple algorithms were successful but not scalable.
- Intelligent Labeling Tools: Today, sophisticated tools leverage machine learning to provide semi-automated and fully automated solutions.
The Role of Machine Learning in Data Annotation
Machine learning enhances the efficiency and accuracy of data annotation processes. Here's how:
1. Automated Image Annotation
Using advanced algorithms, labeling tools can process images and automatically add relevant tags based on learned data patterns. This is particularly useful in industries like autonomous vehicles, where image recognition is critical.
2. Natural Language Processing (NLP)
For text-based data, machine learning models can analyze and categorize vast amounts of text, enabling faster and more accurate labeling of sentiment, topics, and key terms.
3. Quality Assurance
Machine learning-driven labeling tools can continually learn from user feedback, improving accuracy over time. This feature significantly reduces the time spent on quality control and enhances the overall data quality.
Benefits of Using Labeling Tool Machine Learning
The integration of labeling tool machine learning offers numerous benefits, making it an indispensable asset for organizations looking to harness the power of data. Some of these benefits include:
- Increased Efficiency: Automated processes reduce the time required for labeling, allowing teams to focus on analysis and strategy.
- Cost-Effectiveness: Reducing the labor involved in data annotation cuts costs significantly.
- Scalability: Accurate labeling tools adapt easily to varying data sizes and types, providing versatile solutions for growing organizations.
- Consistency: Machine learning models apply the same standards across datasets, enhancing reliability.
Key Features of Modern Labeling Tool Platforms
When selecting a data annotation platform, it’s essential to consider the key features that enhance its functionality:
1. User-Friendly Interface
A straightforward interface ensures that team members can quickly adapt and utilize the tool effectively, minimizing training time.
2. Versatile Annotation Options
Look for tools that offer various annotation types—bounding boxes, segmentation, text tagging, and audio labeling—to cater to different data modalities.
3. Collaboration Features
Platforms that facilitate teamwork enhance productivity, allowing multiple users to work on annotations simultaneously while maintaining version control.
4. Integration Capabilities
Ensure the labeling tool can seamlessly integrate with other data processing and analysis platforms, creating a cohesive workflow from annotation to modeling.
Case Studies: Successful Implementations of Labeling Tool Machine Learning
Real-world applications of labeling tool machine learning demonstrate its transformative impact across various industries:
1. Healthcare Diagnostics
In healthcare, companies like Keylabs.ai have implemented machine learning-based labeling tools to annotate medical images. These tools help in training models that assist in diagnosing conditions with improved accuracy, enabling better patient outcomes.
2. Autonomous Vehicles
Leading automotive manufacturers utilize advanced labeling tools for real-time image processing and object detection. The algorithms trained on accurately annotated images help self-driving cars identify pedestrians, traffic signals, and other vehicles effectively.
3. E-commerce and Retail
E-commerce platforms leverage machine learning for automatic tagging of product images and descriptions. By integrating machine-learning labeling tools, retailers can enhance customer experiences, streamline operations, and provide personalized recommendations.
The Future of Data Annotation and Labeling Tool Machine Learning
As technologies continue to advance, the future of data annotation is poised for significant transformations:
1. Enhanced Precision with AI-driven Annotation
Future tools will leverage deeper learning capabilities, producing annotations that are not just accurate but also contextually relevant.
2. Adaptive Learning Systems
Systems that can adapt to new types of data inputs and learning from their processing will become the norm, decreasing the time needed to retrain models and improving adaptability.
3. Democratization of Machine Learning Tools
As labeling tools become more accessible, small businesses will also harness advanced machine learning technologies, leveling the playing field with larger corporations.
4. Ethical Considerations and Bias Mitigation
As the impacts of AI on society become more scrutinized, the development of labeling tools that mitigate bias in datasets will be crucial for ethical machine learning practices.
Conclusion: Embracing the Change
In the realm of data annotation, the rise of labeling tools powered by machine learning signifies a pivotal change, providing organizations not just with efficiency but with the ability to unlock the full potential of their data. As businesses like Keylabs.ai lead the charge in developing these technologies, the focus must remain not only on speed and accuracy but also on ethical practices and scalability. By embracing these innovations, companies can ensure they remain competitive in an increasingly data-driven world.
In conclusion, the integration of labeling tool machine learning is not just a trend; it is a fundamental shift in how we handle and interpret data, paving the way for a more intelligent, insightful future.