The Importance of Labeling Tools for Machine Learning
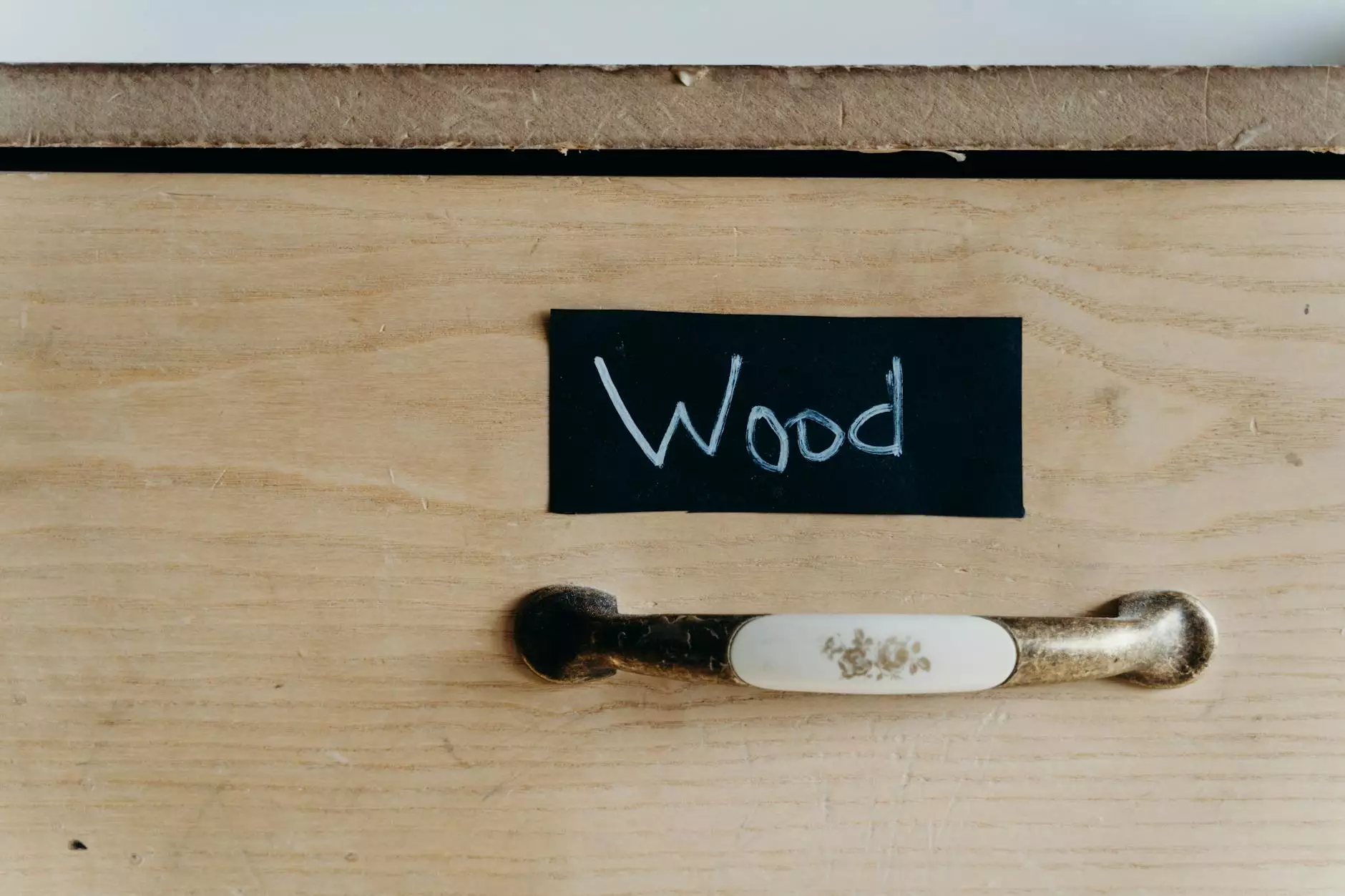
In today's fast-paced digital environment, the ability to harness the power of data has become a critical factor in business success. Businesses are leveraging data analytics to drive strategic decisions, enhance customer experiences, and gain competitive advantages. However, the effectiveness of machine learning models largely hinges on one crucial component: labeling tools for machine learning.
Understanding Data Annotation in Machine Learning
Data annotation is the process of labeling data to enable machine learning algorithms to recognize patterns and make accurate predictions. This foundational work is crucial because machine learning systems learn from this labeled data, making it imperative that the information provided is accurate, rich, and comprehensive.
The Role of Labeling Tools
Labeling tools for machine learning are software applications that assist in the data annotation process. They facilitate the efficient and precise labeling of different types of data, including:
- Images – For tasks such as image classification, object detection, and segmentation.
- Text – For sentiment analysis, named entity recognition, and classification tasks.
- Audio – For speech recognition and sound/event classification.
- Video – For action recognition, tracking, and content moderation.
Why Invest in Quality Labeling Tools?
Investing in high-quality labeling tools can lead to significant advantages for a business. Here are several reasons why:
1. Improved Accuracy
Using sophisticated labeling tools for machine learning enhances labeling accuracy. These tools often come equipped with features that help reduce human error, such as user-friendly interfaces, automated suggestions, and quality assurance mechanisms.
2. Increased Efficiency
The right tools streamline the data annotation process, enabling teams to label large volumes of data quickly. For example, platforms like keylabs.ai provide functionalities that allow for batch processing and parallel annotation efforts, drastically reducing the time required to prepare data for machine learning models.
3. Enhanced Collaboration
Labeling tools facilitate collaboration among data scientists, annotators, and project managers. Through cloud-based solutions, teams can work together in real-time, share progress updates, and ensure that everyone is aligned on project goals and requirements.
Types of Labeling Tools Available
In the market today, there are various types of labeling tools made to cater to differing data types and specific tasks. Here are some prevalent categories:
1. Image Annotation Tools
These tools are specialized for annotating visual content. Common functionalities include bounding boxes, polygons, segmentation, and landmark annotation. They are crucial for training models on tasks such as self-driving cars and facial recognition.
2. Video Annotation Tools
Video annotation tools allow users to annotate clips frame-by-frame. These tools are vital for applications like video surveillance, sports analytics, and autonomous vehicles.
3. Text Annotation Tools
Tools designed for text data often include functionalities for named entity recognition, part-of-speech tagging, and sentiment analysis. These are essential for training NLP models.
4. Audio Annotation Tools
These tools are tailored for tasks like transcribing audio, identifying speaker roles, and labeling sounds. They are particularly useful in speech recognition applications.
Key Features to Consider When Choosing Labeling Tools
When selecting the best labeling tools for your machine learning projects, consider the following features:
1. User-Friendliness
A user-friendly interface is vital to ensure that annotators can effectively carry out their tasks without extensive training. A well-designed tool minimizes the learning curve and enhances productivity.
2. Customization Options
The ability to customize labeling workflows is crucial for organizations with unique needs. Choose tools that allow users to set specific annotation guidelines, define label sets, and integrate with existing workflows.
3. Scalability
As projects grow, so does the amount of data that needs to be labeled. Ensure that your chosen tools can scale accordingly to handle larger datasets without compromising performance.
4. Integration Capabilities
Look for labeling tools that easily integrate with your existing data pipelines and machine learning frameworks. This integration helps maintain a smooth workflow and reduces friction in the data preparation process.
Case Studies: Successful Implementation of Labeling Tools
To illustrate the impact of effective labeling tools, let's look at some case studies of businesses that successfully implemented these tools to enhance their machine learning initiatives.
Case Study 1: Autonomous Driving
A leading automotive company utilized advanced image annotation tools to label thousands of images collected from cameras mounted on vehicles. By employing sophisticated labeling tools, they trained their models to accurately identify pedestrians, road signs, and obstacles. The result was a significant improvement in the safety features of their autonomous vehicles, leading to better customer trust and reduced accident rates.
Case Study 2: Healthcare Diagnostics
A healthcare technology firm leveraged text annotation tools to analyze patient records and clinical notes. By annotating vast amounts of data for medical terms and diagnoses, they developed a machine learning model that could predict potential health risks. The tool enabled clinicians to deliver more personalized care, thereby improving patient outcomes and operational efficiency.
Case Study 3: E-Commerce Recommendation Systems
An e-commerce giant used video annotation tools for product recommendations. By annotating user interaction videos, they identified patterns in customer behavior. The insights gained from this labeled data paved the way for more targeted marketing campaigns, significantly increasing conversion rates and boosting sales.
Conclusion: The Future of Data Annotation and Machine Learning
As machine learning continues to evolve, the importance of labeling tools for machine learning cannot be overstated. They form the backbone of successful machine learning projects by ensuring that data is accurately labeled and ready for model training. Businesses that invest in high-quality, efficient labeling tools, such as those offered by keylabs.ai, are poised to harness the full potential of their data.
In a world where data-driven decision-making is critical, organizations must prioritize data annotation to remain competitive. A robust data annotation strategy, backed by the right tools, will lead to more effective machine learning models, ultimately driving innovation and performance.
Final Thoughts
The future of machine learning hinges on how well organizations manage their data annotation processes. With a plethora of labeling tools for machine learning available today, businesses have a unique opportunity to transform their data into actionable insights. By embracing these technologies, enterprises can remain at the forefront of the AI and machine learning revolution.