Image Annotation for Machine Learning: Unlocking the Power of Data
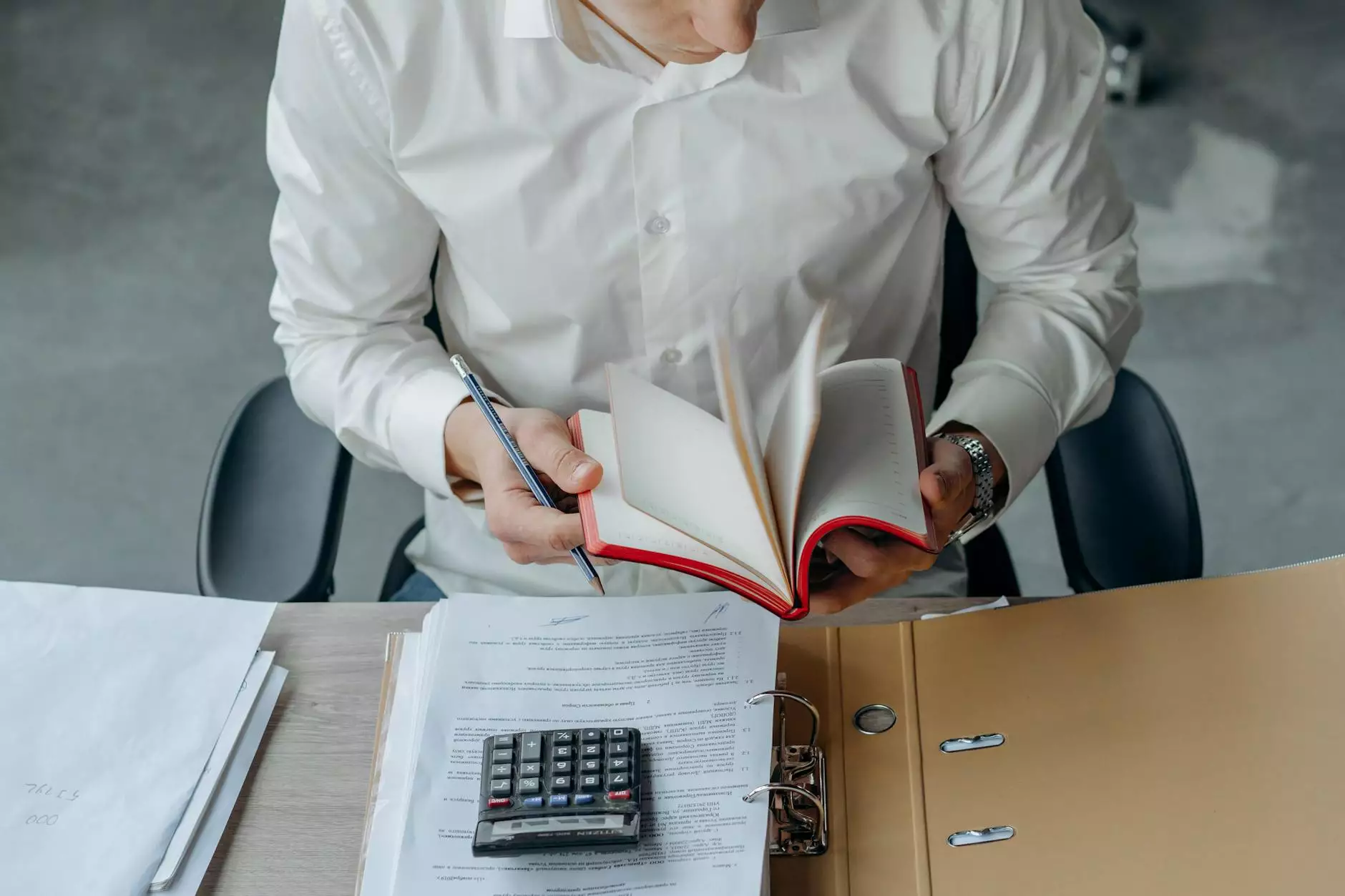
Image annotation for machine learning is a pivotal task that forms the backbone of many innovative technologies today. As artificial intelligence (AI) evolves, the significance of annotated data grows exponentially. This article delves deeply into the world of image annotation, its multitude of applications, the techniques involved, and how businesses can leverage the power of robust data annotation tools and platforms, particularly focusing on what Keylabs.ai offers.
Understanding Image Annotation
Image annotation is the process of labeling images to provide context and meaning to visual data. This can involve identifying objects, understanding scenes, or categorizing images based on predefined criteria.
In the realm of machine learning, specifically in computer vision tasks, annotated images are essential for training algorithms. The more accurately data is annotated, the better the model can learn and generalize from the information presented.
Types of Image Annotation
There are several types of image annotation, each tailored to specific machine learning tasks:
- Bounding Box Annotation: This method involves drawing boxes around objects of interest within an image. It's commonly used in object detection tasks.
- Semantic Segmentation: Here, every pixel in an image is classified into a category, allowing for detailed context understanding.
- Image Classification: Images are sorted into predefined categories, making it a simpler yet vital form of annotation.
- Polygon Annotation: This involves creating polygons around objects, which is beneficial for shapes that aren’t easily defined by rectangles.
- Landmark Annotation: This type focuses on identifying keypoints on objects, such as facial features in an image.
The Importance of Image Annotation in Machine Learning
Annotation acts as the foundation for the supervised learning process, where labeled data is critical for training algorithms. Here are a few reasons why image annotation is vital in machine learning:
- Improved Model Accuracy: Well-annotated images enhance the learning process, which directly impacts the accuracy of predictions and classifications made by AI models.
- Data Diversity: Diverse datasets with varied annotations help models to generalize better, making them robust against different data distributions.
- Automation and Efficiency: With the evolving landscape of machine learning, automated systems benefit greatly from accurate annotated datasets, leading to enhanced operational efficiency.
Applications of Image Annotation
The applications of image annotation are vast and span across various industries:
1. Autonomous Vehicles
In the automotive industry, image annotation for machine learning is critical in developing self-driving cars. Annotated data helps machines understand traffic signs, pedestrians, and obstacles on the road, ensuring safety and efficiency.
2. Healthcare
Medical imaging relies heavily on image annotation. Annotated scans and images allow machine learning models to assist in diagnosing conditions, such as identifying tumors in radiology images.
3. Security and Surveillance
Facial recognition systems leverage annotated images to enhance security measures. Accurate image annotation facilitates better identification and tracking of individuals in real-time.
4. Retail and E-commerce
In retail, image annotation assists in inventory management and personalized marketing strategies by analyzing customer behavior and preferences through visual data.
Keylabs.ai: Your Partner in Image Annotation
Keylabs.ai stands out in the data annotation landscape by providing cutting-edge solutions tailored for various business needs. The platform offers a comprehensive suite of tools designed for effective image annotation for machine learning.
Data Annotation Tool Features
With Keylabs.ai, businesses benefit from a range of features:
- User-Friendly Interface: Designed for ease of use, allowing teams to annotate images efficiently without needing extensive training.
- Scalability: Whether you need to annotate a few images or millions, the platform maintains performance.
- Quality Assurance: Built-in features assure high-quality annotations through validation processes, ensuring data accuracy.
- Collaborative Tools: Teams can work simultaneously, enhancing productivity and enabling real-time feedback.
Data Annotation Platform Benefits
Choosing Keylabs.ai as your data annotation platform offers compelling advantages:
- Cost-Effectiveness: With flexible pricing options, businesses can optimize their budgets while accessing premium features.
- Rapid Turnaround Times: Accelerate your projects with quick annotation processes without sacrificing quality.
- Expert Support: Access to a dedicated team of experts who can assist with complex projects and tailor solutions to specific needs.
- Integration Capabilities: The platform can seamlessly integrate with existing workflows and tools, improving operational efficiency.
Challenges in Image Annotation
While image annotation is critical, it also presents several challenges:
- Complexity of Data: Images can have many objects and layers, making precise annotation difficult.
- Time-Intensive Process: Annotating large datasets can consume significant amounts of time and resources.
- Subjectivity in Annotation: Different annotators may interpret images differently, leading to inconsistencies.
Overcoming Annotation Challenges with Keylabs.ai
Keylabs.ai addresses these challenges through innovative solutions:
By utilizing a combination of automated tools and human expertise, the platform minimizes the challenges associated with data annotation. This hybrid approach ensures that images are accurately labeled while maintaining a swift annotation timeline, ultimately leading to high-quality datasets for machine learning projects.
Future of Image Annotation in Machine Learning
The future of image annotation for machine learning is bright and filled with possibilities:
As machine learning algorithms become more advanced, the demand for high-quality annotated data will grow. Innovations such as deep learning techniques and AI-driven automation will continue to enhance the speed and accuracy of image annotation processes.
Furthermore, the integration of crowdsourcing in annotation tasks will allow businesses to access a larger pool of annotators, improving the diversity and volume of data.
Conclusion
In the ever-evolving landscape of machine learning, accurate and efficient image annotation is crucial. It drives the development of intelligent systems capable of understanding and interacting with the world visually. By partnering with Keylabs.ai, businesses can ensure they harness the power of data through advanced annotation tools and platforms, empowering their machine learning initiatives.
Investing in quality image annotation is not just a necessity—it's a strategic move towards achieving superior machine learning results, ultimately leading to enhanced innovation, better service delivery, and a competitive edge in the market.